As agents take over the discussions, one challenge remains consistent for the businesses building them: how do you effectively monetize agent solutions?
At the core of this shift? Signals.
Signals are a powerful concept that's transforming how AI agent companies measure, demonstrate, and bill for value.
What are signals? The atomic unit of AI work
Signals are (usually) discrete, measurable events that occur when an AI agent performs a meaningful action or reaches a significant milestone.
Think of signals as digital stamps (or breadcrumbs!) that mark each valuable action your AI agent takes.
A signal gets generated whenever an agent:
Completes a specific task
Reaches a checkpoint in a workflow
Produces an output
Interacts with a user or system
Achieves a meaningful outcome
Each signal captures rich metadata:
Timestamp
Agent identifier
Action type
Context (customer ID, conversation details)
Performance data (tokens consumed, processing time, input lengths, costs and margins)
Why we've built our platform around signals
Signals aren't just a technical feature, they’re a business innovation at Paid.
There are 5 key reasons why we’re building them into our core:
1. Bill on what creates value
With signals, you can price and more importantly bill based on:
Individual workflow steps (charge more for high-value steps)
Complete outcomes (only bill when value is delivered)
Volume-based activities (scale with usage)
Hybrid models (combine subscription + outcomes)
The workflow image above shows how each step in a content creation process becomes a billable unit, with distinct pricing tied to the specific value delivered.
2. Transparency and auditability
In a world of AI black boxes, signals create trust. Your customers see exactly what they're paying for.
You can show the actual work performed rather than vague summaries and entitlements.
3. Value-aligned pricing
Signals let you connect pricing directly to business outcomes that matter.
Each meaningful action becomes a distinct signal. In the medical receptionist example above - from appointment scheduling to preventing no-shows.
This creates multiple potential monetization points that your customers would care about.
4. Intelligent Business Decisions
Signal data provide insights:
Which agent actions drive the most customer value?
Where are the performance bottlenecks?
Where are we not monetizing something we could?
How do costs compare to revenue for each action across customers and agents?
5. Evolving Business Models
As your AI capabilities grow, your pricing can evolve without rebuilding your entire infrastructure.
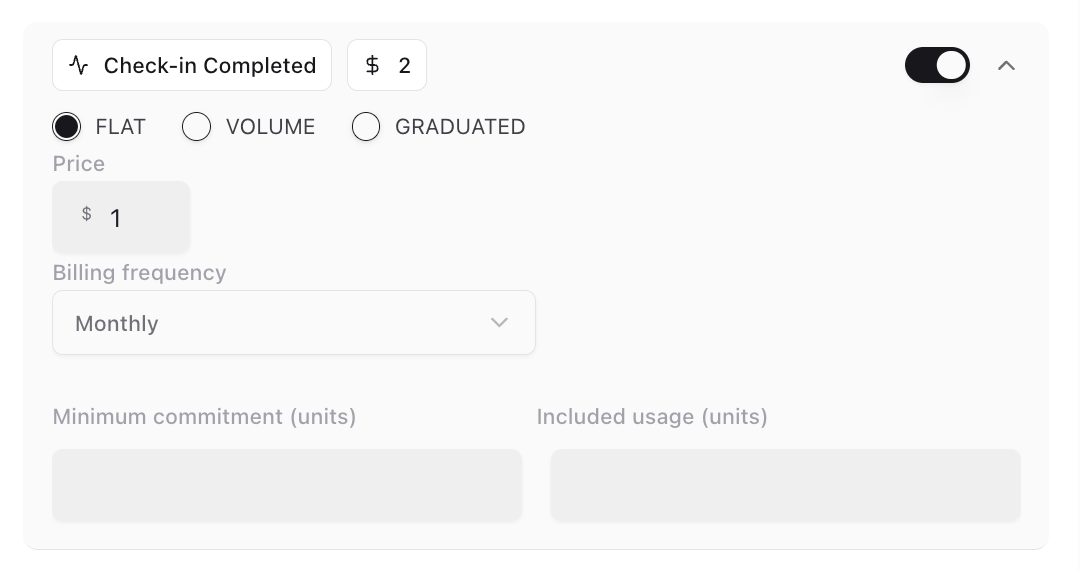
Prepare for the future: Figure out your value signals today
Signals vary across different types of AI agent applications.
I thought I’d give some practical examples of signals to monetize:
AI SDRs / Sales AI agents
Prospecting signals: New lead identified, contact information verified
Outreach signals: Email drafted, email sent, follow-up scheduled
Engagement signals: Response received, meeting requested
Outcome signals: Meeting booked, deal advanced
Document processing agent
Input signals: Document received, format identified
Processing signals: Text extracted, information categorized
Output signals: Summary generated, data entered into system
Quality signals: Accuracy score, confidence level
Customer support agents
Conversation signals: Chat initiated, intent identified
Resolution signals: Answer provided, issue escalated to human
Satisfaction signals: Positive feedback received, problem resolved
Start your signal strategy today
Practically, here are things you can do now:
Map your agent's core value stream - Identify every interaction that creates tangible value
Prioritize high-impact signals - Focus on outcomes that customers would willingly pay for
Design your signal architecture - Create consistent naming and metadata conventions
Start tracking - Ensure signal emission at each critical step
Experiment with pricing models - Test activity-based vs. outcome-based approaches - even in a spreadsheet. Are you leaving money on the table?
The question isn't whether to implement signals-based agent monetization, but how quickly you can make them the backbone of your AI business strategy.
Ready to see it in action? Sign up to our exclusive beta
Innovative thinking to introduce signals. The field of AI is evolving and often different platforms use different terminology like tools or tasks or function calls. Would it make sense to marry signals with traceability logs and evals? Signals should be part of traceability and if eval Metrics don’t hit, don’t charge even if the task was done